Options Volatility Training SVM Operators
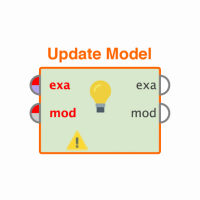

I am new in RapidMiner and trying to run training model traing for historical volatility. I really don't know how to improve the accuracy rate because the highest I can get are only 60.6%. But after adding index series, all of sudden improved to 83.7%. I believe there must be something wrong. Can any expert tell me where I did something wrong because I am trying playing around to improve the accuracy rate for options volatility.
<参数键= " create_label " value = " true " / >
<连接from_port = "训练" to_op = to_port“支持向量机”="training set"/>
Best Answer
-
Thomas_Ott RapidMiner Certified Analyst, RapidMiner Certified Expert, MemberPosts:1,761
Unicorn
I wrote an extensive process on predicting historical volatility for the S&P500 options, with optimization I got around the high 60's, low 70's.
What I see off the bat is that you're using a Dot kernel for the SVM. You'll need to use an RBF kernel and vary the gamma and C values while simultaneously adjust the window traning and testing widths.
0
Answers
Finally can have you here. I did watch all of your video posted previously and very useful to me. Thank you for your advice and will try on it.
Sorry Thomas, If let's say I found a good result, what should I do to predict next 5 days volatility? Could you please further advise?
Yes, you sure can.
Here's a link to my old Blot blog where I auto optimized my Volatility prediction process in RapidMiner and then autogenerated the blog post with images:http://neuralmarket.blot.im/2016-06-06-sandp500-historical-vol-prediction