How to interpret highly significant predictors and low squared correlation?
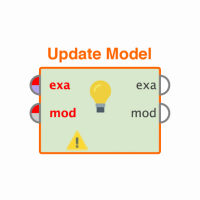

Hello. I did a linear regression analysis on my 42.000 data (online contest results) and after the model building and the model performance calculation, some of my variables turned out to be highly siginificant (4 stars in the tabular view of the model). The p-values were 0,000 for these variables. But then we looked at the squared correlation, and this was low: 0,013. I don't quite understand this contradiction. How can variables be highly significant in predicting the target variable, and the correlation value be very low at the same time? How should I interpret this? Thx in advance!
Tagged:
0
Answers
Well you really don't want your LR model to be built on 'correlated' data and gives rise to the multi-colinearity problem.
Those metrics are really measuring two different theoretical quantities, and thus it is possible to have the problem you describe, even if your predictors are not suffering from multi-collinearity. This is one of the reasons that the entire p-value approach to measuring model effectiveness has come under significant criticism from the Bayesian wing of statistics, because the conventional use and interpretation of p-values can lead to some fairly confusing outcomes.
In classical interpretation, the p-value is a measure used to evaluate the null-hypothesis (how likely is the observed effect to be seen across repeated samples given the truth of no effect), given the underlying assumptions about the shape of the distributions being sampled (normal, non-heteroschedastic, etc.). Thus, because of the properties of thsoe distributions, with very large sample sizes, it is possible to get low p-values for a large number of effects, even if those effects are actually quite small, and the overall model fit is quite poor (as evidenced by your low R2). In the era of big data, this problem has become even more commonplace, and thus the criticisms of this approach to model building and interpretation have gained ground.
Personally I would focus more on the outcomes of the model and its potential use in the actual business case and less on the p-values of individual coefficients---or switch to a modeling approach that is not primarily based on interpreting p-values at all.
Lindon Ventures
Data Science Consulting from Certified RapidMiner Experts