"How to test the assumptions of logistic regression in RM?"
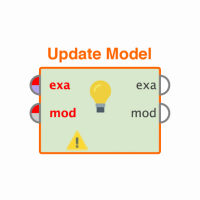

Hello ! I am getting a bit lost in my analysis and I am stuck between two steps of my methodology. I had aclassificationproblem (churn) with a dataset of100variables for 100 000 examples and, after removing attributes with too many missing values and those that were too correlated to others (pairs with a correlation above 75%) or with a too small variance, I have46 attributesand80 000examples in my training set.
However, I'd like to have between10-15 attributes大约同时,我我们ing abackward elimination. This means that I have to alreadychoose a predictive modeland for now, I chose eitherRandom ForestorLogistic Regression. My first question would be: which backward elimination model choosing knowing that the results are much different whether I do it with a logistic regression or with Random Forest? Then, I have mainly 2 questions:
1. ASSUMPTIONS BEHIND MODELS
For logistic regression, if I choose this one, I am not sure how to verify the assumptions linked to this model in Rapid Miner:
2. CHOOSING THE MODEL - Blackbox models
Finally, after the backward elimination, how can I choose the best model? I know I can compare their ROC, AUC or accuracy but I also need to take into accountwhether the hypothesis are verifiedandwhether it's easily interpretable for anyone not familiar with data science. For example, I would like to avoid so-called "blackbox" models such as Neural Nets but would you consider Random Forest as a blackbox model?
Thank you all for your help, I'm getting a bit stressed by my incoming deadline and will appreciate all advices
However, I'd like to have between10-15 attributes大约同时,我我们ing abackward elimination. This means that I have to alreadychoose a predictive modeland for now, I chose eitherRandom ForestorLogistic Regression. My first question would be: which backward elimination model choosing knowing that the results are much different whether I do it with a logistic regression or with Random Forest? Then, I have mainly 2 questions:
1. ASSUMPTIONS BEHIND MODELS
For logistic regression, if I choose this one, I am not sure how to verify the assumptions linked to this model in Rapid Miner:
- I have no idea how to test in Rapid Miner thelinearity between the independent variables and the log odds of the dependent variable. I read a bit about Box-Tidwell test but can't find that on RM. Do you have any advices or help to offer?
- Hypothesis ofLittle or no multicolinearity among the variables: is it problematic as I know some are still correlated above 50%?
2. CHOOSING THE MODEL - Blackbox models
Finally, after the backward elimination, how can I choose the best model? I know I can compare their ROC, AUC or accuracy but I also need to take into accountwhether the hypothesis are verifiedandwhether it's easily interpretable for anyone not familiar with data science. For example, I would like to avoid so-called "blackbox" models such as Neural Nets but would you consider Random Forest as a blackbox model?
Thank you all for your help, I'm getting a bit stressed by my incoming deadline and will appreciate all advices

Tagged:
0
Answers
as a data scientist you may not be intersted if your assumptions are valid (it might be good though). You are interested in choosing the model which can optimize your quality measure (e.g. revenue). If a model like a linear regression, which is only valid for linear cases works, than i take it.
BR,
Martin
Dortmund, Germany
Ok, thanks for the reassuring reply! It's for my thesis though and I don't know how much they'll pay attention to that. My jury is not composed of statistical experts, which is nice, but I still fear that using a model that does not allow multicollinearity between variables may look bad. But I guess I'll leave the linearity issue on the side then!
Regarding independency between variables, would you consider a correlation of 50% as breaking the independency? I tried remove all pairs above that threshold.
Thanks again!
Ophélie
i would just test what threshold is better and treat it as a hyperparameter of the modelling process.
Best,
Martin
Dortmund, Germany
Lindon Ventures
Data Science Consulting from Certified RapidMiner Experts
CHOOSING THE MODEL:
You can also explain neural networks. First thing with the dataset (Classification) is to visualize the class distributions. Try to visualize the the dataset using T-SNE in 2 or 3 dimension. This is will give you a better understanding of how your classes are distributed. As all the algorithms doesn't give us best results we can observe and hypothesize based on the distributions. For example: If the classes are plotted as small distributions here and there on the plot, neural networks and deep learning works better. This is because they are able to calculate local minima of each distribution. If the classes are linearly separated then traditional algorithms work better. For subset selection, you can use best subset selection which is computationally expensive but gives the best attributes that are significant for training and testing based on the 2^p model training. This will give you the significant attributes when you use 10 variables or 15 variables similar to step wise selection. Lasso can be used for multicollinearity problem.
Two more performance metrics that needs to be considered are Kappa(inter-rater agreement) and RMSE which gives you the performance based on individual class prediction. Accuracy cannot be good in all cases as it depends on class distribution (class imbalance).
@mschmitzcorrect me if there is any issue in this.
Thanks,
Varun
Varun
https://www.varunmandalapu.com/
Be Safe. Follow precautions and Maintain Social Distancing
Lindon Ventures
Data Science Consulting from Certified RapidMiner Experts