Auto model and variables quality
Hi there,
What is the logic behind assigning yellow / green status to variables in Auto Model?
I just came across the situation where variables with higher stability and ID-ness are considered green, while those with lower stability / ID-ness are yellow. I would expect it to be the other way around.
What is the logic behind assigning yellow / green status to variables in Auto Model?
I just came across the situation where variables with higher stability and ID-ness are considered green, while those with lower stability / ID-ness are yellow. I would expect it to be the other way around.
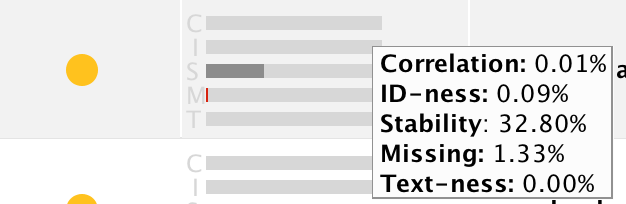
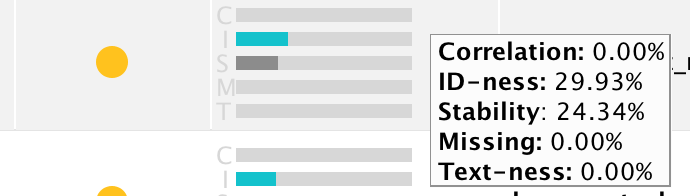
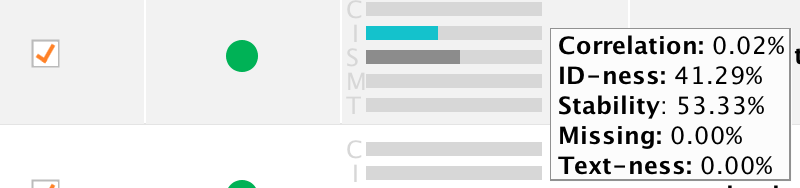
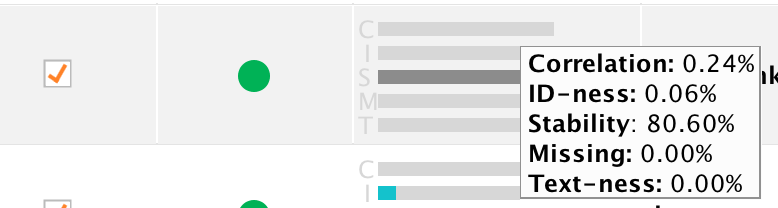
Tagged:
0
Best Answer
-
IngoRM Administrator, Moderator, Employee, RapidMiner Certified Analyst, RapidMiner Certified Expert, Community Manager, RMResearcher, Member, University ProfessorPosts:1,751
RM Founder
Hi,The first two cases are yellow because of a close-to-zero correlation with the label. The full logic is actually described in the help text of Auto Model. Here are the key points (from 9.2 Beta):
Status
The colored status bubble provides a quality indicator for a data column.
- Red:A red bubble indicates a column of poor quality, which in most cases you should remove from the data set. Red can indicate one of the following problems:
- More than 70% of all values in this column aremissing,
- The column is practically anIDwith (almost) as many different values as you have rows in your data set but doesnotlook like a text column at the same time (see below),
- The column is practically constant, with more than 90% of all values being the same (stable), or
- The column has a correlation of lower than 0.0001% or higher than 95% with the label to predict (if a label is existing).
- Yellow:A yellow bubble indicates a column which behaves like an ID but also looks like a text or which has either a very low or a very high correlation with the target column. They correlation-based yellow bubbles can only appear if the task is "Predict".
- ID which looks like text:this column has a high ID-ness and would be marked as red but at the same time has a text-ness of more than 85%.
- Low Correlation:a correlation of less than 0.01% indicates that this column is not likely to contribute to the predictions. While keeping such a column is not problematic, removing it may speed up the model building.
- High Correlation:a correlation of more than 40% may be an indicator for information you don't have at prediction time. In that case, you should remove this column. Sometimes, however, the prediction problem is simple, and you will get a better model when the column is included. Only you can decide.
I think the only missing number is the ID-ness threshold for becoming red. This threshold actually depends on the type of the column and is 0.7 for nominal columns and 0.99 for integer columns.
Hope this helps,Ingo
7 - Red:A red bubble indicates a column of poor quality, which in most cases you should remove from the data set. Red can indicate one of the following problems:
Answers
Vladimir
http://whatthefraud.wtf
@kypexin@IngoRM,我认为这是一个很好的考虑。我注意到a similar "why this color" as I considered some columns as to be important prior to any modeling. I propose to use some standard datasets with the knowledge of domain expertise to demonstrate the impact of following the full logic for all datasets. In other words it would be nice to find examples where some pitfalls could be illustrated.
It is a question that comes from the audience when the steps of automodel are demonstrated.
Cheers
Sven
Constructive and realistic feedback. Balancing between Automodel and #noblackboxes is essential and "the traffic lights are a guidance" is the answer.
Cheers
Sven