虽然训练示例集和真实数据集是相同的,但在应用模型时缺少属性
你好,
我是新的rapidMiner,我试图分类飞YouTube评论创新产品到客户需求或不是。乐鱼官网手机版下载
这两个示例集应该与我使用来自培训数据的单词列表并将其应用到我想用来自数据操作符的流程文档进行分类的数据相同。在下面的图片中,你可以看到两个数据集的比较。
我使用RapidMiner自动模型创建一个支持向量机分类过程,然后我用这个过程存储模型。然后我使用以下过程:
.
.
.
. class="generate_empty_attribute" compatibility="9.3.001
.
.
.
我还附上了100行样本数据。如果这个问题已经解决了(找不到对我的情况有用的东西),或者如果我犯了一些简单的错误,我道歉。
如果有人知道如何纠正英语拼写,(我已经尝试使用快速矿工社区发布的textblob python脚本。它会改变已经正确的单词,例如“Big”变成“Fig”)我也会非常感激。
提前谢谢,
田纳西州
我是新的rapidMiner,我试图分类飞YouTube评论创新产品到客户需求或不是。乐鱼官网手机版下载
这两个示例集应该与我使用来自培训数据的单词列表并将其应用到我想用来自数据操作符的流程文档进行分类的数据相同。在下面的图片中,你可以看到两个数据集的比较。
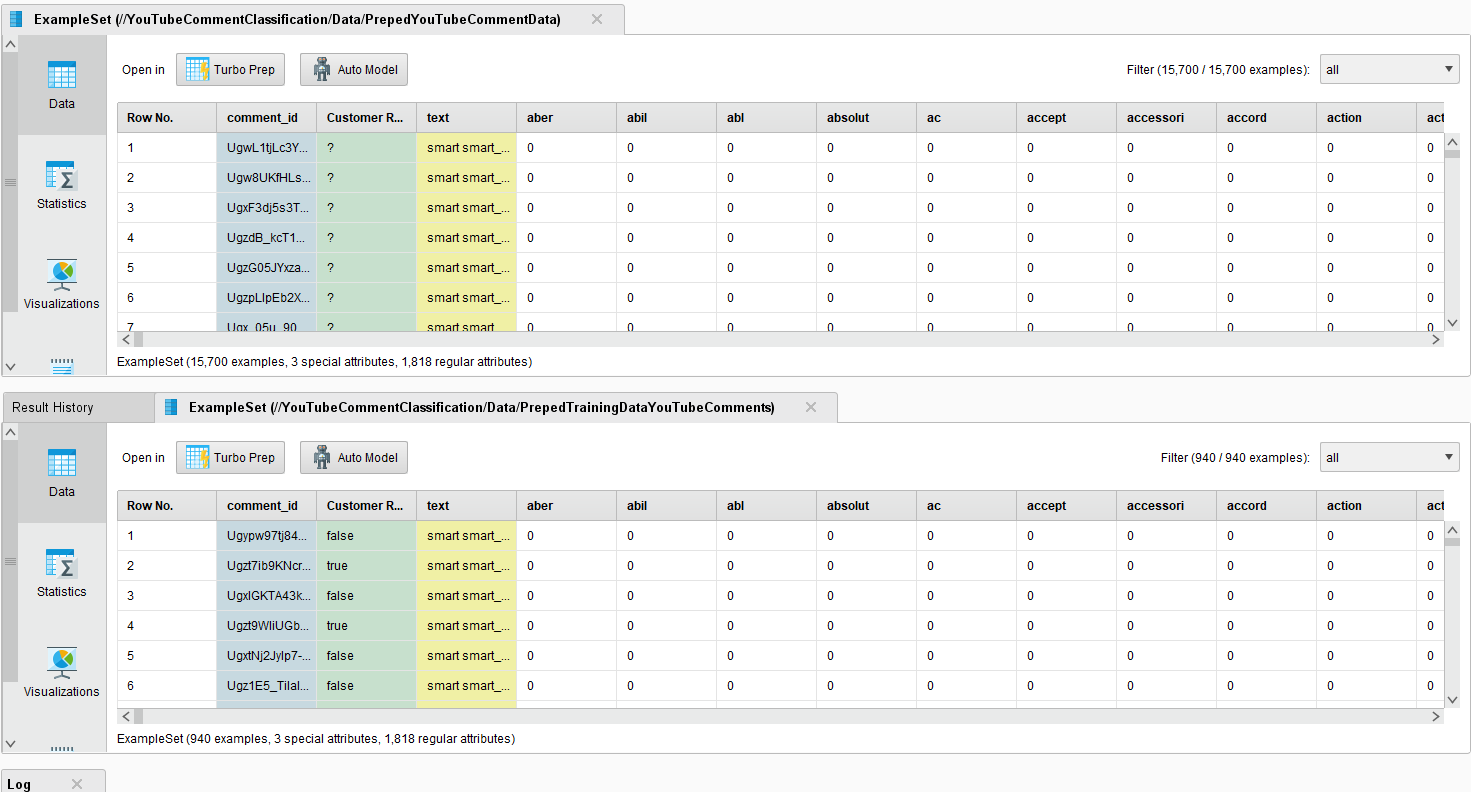
我使用RapidMiner自动模型创建一个支持向量机分类过程,然后我用这个过程存储模型。然后我使用以下过程:
<?xml version = " 1.0 " encoding = " utf - 8 " ?> <过程version = " 9.3.001”>
> <上下文
<输入/ >
<输出/ >
<宏>
宏观> <
<键>文本关键> < /
让我们试试非常简单的方法。我喜欢智能手表
宏观> < /
< /宏>
> < /上下文
<参数键= " logverbosity " value = " init " / >
<参数键= " random_seed " value = " 2001 " / >
<参数键= " send_mail " value = "永远" / >
<参数键= " notification_email“价值= " / >
<参数键= " process_duration_for_mail " value = " 30 " / >
<参数键=“编码”值= "系统" / >
<过程扩展= " true " >
<参数键= " repository_entry " value = " . . /数据/ PrepedYouTubeCommentData " / >
< /操作符>
<参数键= " repository_entry " value = " . . /结果/ SvmClassificationYComments " / >
< /操作符>
<列出关键= " application_parameters " / >
<参数键= " create_view " value = " false " / >
< /操作符>
<参数键= " attribute_filter_type " value = " numeric_value_filter " / >
<参数键= "属性" value = " / >
<参数键= "属性" value = " / >
<参数键= " use_except_expression " value = " false " / >
<参数键= " value_type " value = " attribute_value " / >
<参数键= " use_value_type_exception " value = " false " / >
<参数键= " except_value_type " value = "时间" / >
<参数键= " block_type " value = " attribute_block " / >
<参数键= " use_block_type_exception " value = " false " / >
<参数键= " except_block_type " value = " value_matrix_row_start " / >
<参数键= " numeric_condition " value = "祝辞0 " / >
<参数键= " invert_selection " value = " false " / >
<参数键= " include_special_attributes " value = " false " / >
< /操作符>
/> .
> < /过程
< /操作符>
> < /过程
然而,我总是得到这个错误:
我使用youTube上的教程和视频类VbNhvYQZ2v0以及rapidMiner学院的TextMining和机器学习课程来构建我的过程。
这个过程显示了我对训练数据的预处理:
.
.
.
.
. class="set_role" compatibility="9.3.001
.
这个过程显示了我想分类的数据的预处理:
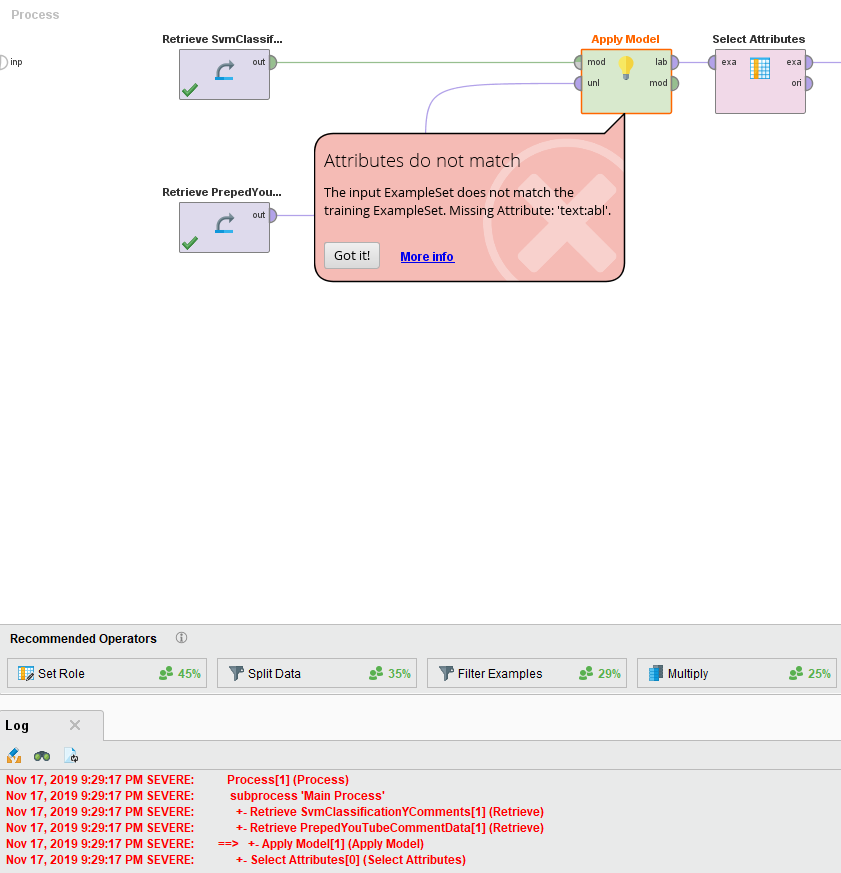
我使用youTube上的教程和视频类VbNhvYQZ2v0以及rapidMiner学院的TextMining和机器学习课程来构建我的过程。
这个过程显示了我对训练数据的预处理:
<?xml version = " 1.0 " encoding = " utf - 8 " ?> <过程version = " 9.3.001”>
> <上下文
<输入/ >
<输出/ >
<宏/ >
> < /上下文
<参数键= " logverbosity " value = " init " / >
<参数键= " random_seed " value = " 2001 " / >
<参数键= " send_mail " value = "永远" / >
<参数键= " notification_email“价值= " / >
<参数键= " process_duration_for_mail " value = " 30 " / >
<参数键=“编码”值= "系统" / >
<过程扩展= " true " >
<参数键= " repository_entry " value = " . . /数据/ SampleDataYouTubeComments " / >
< /操作符>
<参数键= =“子集”/“attribute_filter_type”值>
<参数键= "属性" value = "评论" / >
<参数键= "属性" value = " |评论| product_name | product_type " / >
<参数键= " use_except_expression " value = " false " / >
<参数键= " value_type " value = "名义" / >
<参数键= " use_value_type_exception " value = " false " / >
<参数键= " except_value_type " value = " file_path " / >
<参数键= " block_type " value = " single_value " / >
<参数键= " use_block_type_exception " value = " false " / >
<参数键= " except_block_type " value = " single_value " / >
<参数键= " invert_selection " value = " false " / >
<参数键= " include_special_attributes " value = " false " / >
< /操作符>
<参数键= " create_word_vector " value = " true " / >
<参数键= " vector_creation " value = " TF-IDF " / >
<参数键= " add_meta_information " value = " true " / >
<参数键= " keep_text " value = " true " / >
<参数键= " prune_method " value = "绝对" / >
<参数键= " prune_below_percent " value = " 3.0 " / >
<参数键= " prune_above_percent " value = " 30.0 " / >
<参数键= " prune_below_absolute " value = " 2 " / >
<参数键= " prune_above_absolute " value = " 1000 " / >
<参数键= " prune_below_rank " value = " 0.05 " / >
<参数键= " prune_above_rank " value = " 0.95 " / >
<参数键= " datamanagement " value = " double_sparse_array " / >
<参数键= " data_management " value = "自动" / >
<参数键= " select_attributes_and_weights " value = " false " / >
<列出关键= " specify_weights " / >
<过程扩展= " true " >
<参数键= "字符" value = ": " / >
<参数键= "语言" value = "英语" / >
<参数键= " max_token_length " value = " 3 " / >
< /操作符>
< /操作符>
<参数键= " max_length " value = " 2 " / >
< /操作符>
<参数键= " min_chars " value = " 2 " / >
<参数键= " max_chars " value = " 25 " / >
< /操作符>
< portSpacing端口= " source_document”间隔= " 0 " / >
> < /过程
< /操作符>
<参数键= " repository_entry " value = " . . /结果/ WordlistForCR " / >
< /操作符>
<参数键= " attribute_filter_type " value = "单一" / >
<参数键= "属性" value = " / >
<参数键= " use_except_expression " value = " false " / >
<参数键= " value_type " value = "数字" / >
<参数键= " use_value_type_exception " value = " false " / >
<参数键=“except_value_type”值= "真正的" / >
<参数键= " block_type " value = " value_series " / >
<参数键= " use_block_type_exception " value = " false " / >
<参数键= " except_block_type " value = " value_series_end " / >
<参数键= " invert_selection " value = " false " / >
<参数键= " include_special_attributes " value = " false " / >
< /操作符>
<参数键= " attribute_filter_type " value = "单一" / >
<参数键= "属性" value = " / >
<参数键= " use_except_expression " value = " false " / >
<参数键= " value_type " value = " attribute_value " / >
<参数键= " use_value_type_exception " value = " false " / >
<参数键= " except_value_type " value = "时间" / >
<参数键= " block_type " value = " attribute_block " / >
<参数键= " use_block_type_exception " value = " false " / >
<参数键= " except_block_type " value = " value_matrix_row_start " / >
<参数键= " invert_selection " value = " false " / >
<参数键= " include_special_attributes " value = " false " / >
<列出关键= " value_mappings " >
<参数键= " 1 " value = " true " / >
<参数键= " 0 " value = " false " / >
< / >列表
<参数键= " consider_regular_expressions " value = " false " / >
<参数键= " add_default_mapping " value = " false " / >
< /操作符>
<参数键= " target_role " value = "标签" / >
<列出关键= " set_additional_roles " >
<参数键= " comment_id " value = " id " / >
< / >列表
< /操作符>
<参数键= " repository_entry " value = " . . /数据/ PrepedTrainingDataYouTubeComments " / >
< /操作符>
> < /过程
< /操作符>
> < /过程
这个过程显示了我想分类的数据的预处理:
<?xml version = " 1.0 " encoding = " utf - 8 " ?> <过程version = " 9.3.001”>
> <上下文
<输入/ >
<输出/ >
<宏/ >
> < /上下文
<参数键= " logverbosity " value = " init " / >
<参数键= " random_seed " value = " 2001 " / >
<参数键= " send_mail " value = "永远" / >
<参数键= " notification_email“价值= " / >
<参数键= " process_duration_for_mail " value = " 30 " / >
<参数键=“编码”值= "系统" / >
<过程扩展= " true " >
<参数键= " repository_entry " value = " . . /结果/ WordlistForCR " / >
< /操作符>
<参数键= " repository_entry " value = " . . /数据/ DataYouTubeComments " / >
< /操作符>
<参数键= "属性" value = " |评论| product_name | product_type " / >
<参数键= " use_except_expression " value = " false " / >
<参数键= " value_type " value = "名义" / >
<参数键= " use_value_type_exception " value = " false " / >
<参数键= " except_value_type " value = " file_path " / >
<参数键= " block_type " value = " single_value " / >
<参数键= " use_block_type_exception " value = " false " / >
<参数键= " except_block_type " value = " single_value " / >
<参数键= " invert_selection " value = " false " / >
<参数键= " include_special_attributes " value = " false " / >
< /操作符>
<参数键= " create_word_vector " value = " true " / >
<参数键= " vector_creation " value = " TF-IDF " / >
<参数键= " add_meta_information " value = " true " / >
<参数键= " keep_text " value = " true " / >
<参数键= " prune_method " value = "没有" / >
<参数键= " prune_below_percent " value = " 3.0 " / >
<参数键= " prune_above_percent " value = " 30.0 " / >
<参数键= " prune_below_rank " value = " 0.05 " / >
<参数键= " prune_above_rank " value = " 0.95 " / >
<参数键= " datamanagement " value = " double_sparse_array " / >
<参数键= " data_management " value = "自动" / >
<参数键= " select_attributes_and_weights " value = " false " / >
<列出关键= " specify_weights " / >
<过程扩展= " true " >
<参数键= "字符" value = ": " / >
<参数键= "语言" value = "英语" / >
<参数键= " max_token_length " value = " 3 " / >
< /操作符>
< /操作符>
<参数键= " max_length " value = " 2 " / >
< /操作符>
< portSpacing端口= " source_document”间隔= " 0 " / >
> < /过程
< /操作符>
<参数键= " value_type " value = "多项式" / >
< /操作符>
<参数键= " attribute_filter_type " value = "单一" / >
<参数键= "属性" value = " / >
<参数键= " use_except_expression " value = " false " / >
<参数键= " value_type " value = " attribute_value " / >
<参数键= " use_value_type_exception " value = " false " / >
<参数键= " except_value_type " value = "时间" / >
<参数键= " block_type " value = " attribute_block " / >
<参数键= " use_block_type_exception " value = " false " / >
<参数键= " except_block_type " value = " value_matrix_row_start " / >
<参数键= " invert_selection " value = " false " / >
<参数键= " include_special_attributes " value = " false " / >
<列出关键= " value_mappings " >
<参数键= " 1 " value = " true " / >
<参数键= " 0 " value = " false " / >
< / >列表
<参数键= " consider_regular_expressions " value = " false " / >
<参数键= " add_default_mapping " value = " false " / >
< /操作符>
<参数键= " target_role " value = "标签" / >
<列出关键= " set_additional_roles " >
<参数键= " comment_id " value = " id " / >
< / >列表
< /操作符>
<参数键= " repository_entry " value = " . . /数据/ PrepedYouTubeCommentData " / >
< /操作符>
/> .
> < /过程
< /操作符>
> < /过程
我还附上了100行样本数据。如果这个问题已经解决了(找不到对我的情况有用的东西),或者如果我犯了一些简单的错误,我道歉。
如果有人知道如何纠正英语拼写,(我已经尝试使用快速矿工社区发布的textblob python脚本。它会改变已经正确的单词,例如“Big”变成“Fig”)我也会非常感激。
提前谢谢,
田纳西州
标记:
0
最佳答案
-
田纳西州 成员职位:5
新手
我把支持向量机模型操作符从自动模型过程中复制到交叉验证中并创建了另一个模型。在这个模型中,我可以输入需要分类的数据,而不会得到错误消息。现在它工作得很顺利。但还是浪费了3天。
因此,我建议如果您对automodler创建的模型有问题,将模型复制到交叉验证中。0
答案
斯科特
Lindon合资企业
乐鱼平台进入来自认证RapidMiner专家的数据科学咨询
提前谢谢,
田纳西州
我使用rapidminer automodell创建这个模型,它甚至不能除了自己的训练数据。好像出了什么问题。我将尝试手动创建模型。