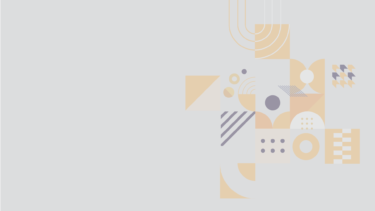
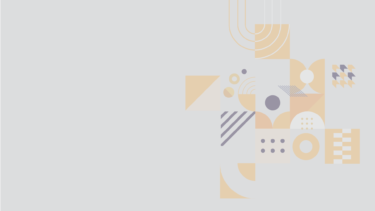
使用RapidMiner进行基于r的需求预测
用高度准确、高度可扩展的需求预测改进供应链
由Dominos数据科学经理Ryan Frederick介绍乐鱼平台进入
对于一个以可靠的服务和快速的产品交付为傲的组织来说,预测整个供应链的需求是至关重要的。看看Domino的数据科乐鱼平台进入学团队如何应对这一挑战,如何完成复杂的时间序列预测练习(从原型到交付),并发现了一种创新的方法来扩展基于r的时间序列模型,以减少错误和加快运行时。
观看下面的完整视频,了解Dominos的数据科学团队如何使用RapidMiner通过可扩乐鱼平台进入展的时间序列预测和基于伸缩r的模型来改善供应链。
00:04好。谈谈我自己。我是达美乐的数据科学经理。乐鱼平台进入我是第一次参加智慧大会。所以很明显,我同意在没有任何背景的情况下进行演讲。我以前的一位导师曾对我说:“要害怕想要拿麦克风的人。”显然,我今天就是那个人。所以事后,私下里,谁来告诉我我做得怎么样,好吗?我说得够多了。达美乐是美国市场占有率第一的披萨公司。 The road to number one is filled with technology innovations that I think probably many of you are familiar with. You will have potentially seen some of the marketing around our mobile app. So there were times when the mobile app can do voice recognition and image recognition. We had a project where we would allow a loyalty customer to take a picture of any pizza and earn 10 loyalty points. So that’s the kind of innovation we’re doing. It could have been a picture of a dog toy, and it would still– a pizza-shaped dog toy, and it would still work. So it’s with that kind of focus on innovation that I come to talk to you today about supply chain demand forecasting. Fun.
01:23所以我的项目目标是为我的客户,也就是供应链,提供高度准确,高度可扩展的需求预测。问题是整个生态系统都有共享的资源,而且生态系统正在迅速扩张。乐鱼体育安装我们中的很多人都在用固定的资源做数据科学。乐鱼平台进入乐鱼体育安装所以我要告诉你们的解决方案是将我使用的时间序列预测工具扩展并创造性地思考以保持较小的占用以免影响到我的一些同行。核心是,我们讨论的是商店库存生命周期,对吧?这始于你们这些饥饿的顾客,他们点了食物,耗尽了商店里的库存。它由商店经营者负责,在一天结束时,他们负责清点库存。他们通过在线工具订购库存。我们的供应链系统随后出现,他们满足库存需求,重新补充商店的库存。正是这个商店操作的过程抛出了大量的数据供我们分析。 So that’s where we’ll be mining insights.
02:37我们的目标是,高度准确,高度可扩展的需求预测。一个简单的例子。我不能给任何人他们会用来逆向工程我的东西的信息。所以你得到了一张奶酪需求和磅数的图表,没有斧头和日期。蓝线显然代表这一系列商店的奶酪需求历史,然后红色虚线是我们的预测。你可以看到一些重要的数据点,它们用灰色条表示。所以我想确切地告诉你们这意味着什么,但这可能是一个重要的日历事件。一年中的某些日子,人们会点更多的披萨,这也可能是一种全国性的促销。我们为什么要这么做呢?业务价值来自于我们从预测中得到的信息,所以我们可以给我们的供应商一个即将到来的需求提振的预警。 Nobody likes to be shocked with large demand and have to figure out where to source the product from, so we give our suppliers heads up. It gives us the option to reduce food waste. So in the stores and in the supply chain centers, let’s optimize against food waste. And lastly, we can scale demand to meet the demand, right? So if it’s going to be a lower volume week, then maybe we don’t need as many folks producing dough.
03:50这就是商业价值的来源。你们都知道这对销售C层套件有多重要,如何让你的产品运转起来。那么我们要如何解决这个问题呢?达美乐有很多可用的资源。乐鱼体育安装我所在的团队大约有50人。我没有管理所有的50个。我只能勉强应付。但该团队中有许多人拥有高等学位。顺便说一下,我这张幻灯片的观点是,我们有很多方法可以解决这个问题,我将给你们展示我们所做的。我们的员工拥有化学、计算机科学、应用统计学和电子工程等专业的高等学位。 One guy has three masters, one of which is nuclear science, some talented people. And then we have a comprehensive text act, which kind of touches on the user’s desktop environment, an AI/ML server side environment where you can run RapidMiner, JupyterHub, RStudio. We’ve got a couple of Nvidia GPU servers in our database at the bottom there with SQL and Hadoop. Most importantly, the RapidMiner SAC. And this is going to be principal to a number of the techniques I talk about. We have three queues, so if you have used RapidMiner server, you know what a queue is. We have three of them kind of named after who pays for it. But I have access to use any of them when I need to. And each queue has 2 machines underneath with 40 cores on the data science queue, 40 on the marketing, and 80 on the memory queue. So these are my tools.
05:16原型,我们开始的地方,并不是让你读每一个过程,它的概念是查询SQL Server数据库,接收模型所需的输入。我待会再解释。将数据传递到模型中,运行建模拟合和预测,然后将结果写入它们需要去的地方,即某些下游生产系统。如果你是程序员,请举手。也许你应该坐在前面。关于R-script,我只简单地讲一下因为不是每个人都是程序员。这里的想法是从数据库中接收三个信息顺便说一下,我们使用的是Facebook的开源时间序列预测工具Prophet。所以Prophet需要大量的输入。RapidMiner接收到的SQL查询将示例集传递给预测函数。我们将其过滤到一个单一的SKU供应链中心组合。 So think Michigan cheese or Georgia pepperoni filtering down to just one thing. We run fit in forecast, and then we wrap that whole thing up with a parallel process, and ours do parallel package, so we can do 16 scenarios concurrently.
06:32改进的时间线,这个东西还在生产中,它挺过了许多数据科学项目遇到的巨大障碍。乐鱼平台进入Ingo今天早上提到,只有不到1%的项目最终能够投入生产。我是这样做的。RapidMiner并非对每一块都不可或缺。我将专注于它所在的地方,只是一些关于每个里程碑意味着什么的高层次思考。首先,我们在单个VM上启动了我们的原型,回想一下RapidMiner架构。我们要求它做200个预测,它花了8个小时。有人对8小时2个200的预测满意吗?所以我们做的第一件事是看看为什么要花这么长时间,大部分时间只是从数据库中检索数据。数据工程解决了这个问题。 We got down to one VM, 200 forecasts in 15 minutes. That’s a lot more interesting. So then the business said, “Great, you’re getting some performance from runtime perspective. How about model performance?” So we took the original model, and I’ll get into this in a minute. We did some grid search in Bayesian optimization to replace the default, the Facebook profit defaults. That took our MAPE from 6.5% to 6.23%, so we got a nice little boost from simply training hyperparameters. And the business said, “Hey, this is great. Okay. You’ve been doing a pilot set of inventory items. Let’s do them all.” That meant a 20X increase in terms of what they’re asking the workload to do. So my runtime went to a now regrettable, one VM, 4,000 forecasts, eight hours again. We’re back to eight hours, and the data footprint was over 150 gigabytes on the disk. So also, not good because my database is limited in size and I need to shrink the footprint.
08:25回到数据工程。我们使用聚集的comps存储索引。如果你不知道那是什么,不用担心。结果是我们的数据占用减少到5gb。所以我们解决了规模扩张的问题,但我们仍然要忍受8小时的运行时间。除了让它跑得更快,你还能做什么?你可以要求它早点开始。我们的大部分工作,都是安排在凌晨4点开始或者我们预计没人上班的时候。我构建了一个小的RapidMiner过程,使其基于事件。所以它只是检验。 Are all predecessors done? Are all predecessors done? And the second they are, then it kicks off. So I saved myself 15, 20 minutes. Big win there. And then I’ll end on two things that I haven’t done yet, but we’re soon going to do, which will get us down to where we’re going. And that’s we’re going to use all six of the VMs. We’re going to do 4,000 forecasts in 27 minutes. Remember where we started. 200 forecasts, eight hours, so we’re much faster and a huge volume more of forecasts to do.
09:30现在我要让你们注意到RapidMiner在解决方案中是不可或缺的。首先,我们想要调整这些超参数,以便业务能够适应准确性。我用你们之前见过的函数,把它参数化,对吧?我只是说,“让我们允许默认变量移动一个移位,我们将通过一个所谓的随机网格搜索列表的场景来测试。”如果我在单个VM上运行它,它将花费60小时,而我不想等待60小时。我想明天看到结果。这就是我入侵RapidMiner来做我想做的事情的地方,也就是并行并行处理。我就是这么称呼它的。这里的想法是我们有一个循环,一个子进程和六个调度进程,它们简单地指向我们有两次的每个RapidMiner队列。分配工作的方式是当监听器得到第一个作业时,它把它发送给第一个机器,几毫秒后,第二个作业到达监听器,它把它发送给另一个机器。 So I’m taking my workload from running on one machine to now splitting it across six. So this is a little trick there with schedule process.
10:46一旦网格搜索完成,我们使用网格搜索结果作为所谓贝叶斯优化的种子。再次强调,我们所做的就是取已有的函数将其中的某些部分参数化然后调用r -包称为r -贝叶斯优化,这在一定程度上平衡了寻找参数中的热点和搜索未搜索区域的需要。我先停一下。昨天有人去参加黑客马拉松了吗?我从中学到的一点是,低代码可能比这么多代码更好。我的家庭作业之一就是弄清楚如何在原生RapidMiner函数中做这类事情。那么如何利用网格搜索和贝叶斯优化呢?我已经知道答案了。MAPE从6.5%提高到6.23%。我们使用自己的hack在不同的机器上安排子过程,它花了10个小时而不是60个小时。 So the next day, I had the results ready for analysis. And for any of you who are sitting in the front row, you might be able to read the grid on the right, which is nothing more than a list of all the scenarios we tested iterating over those default parameters. And you’ll see that the R-Bayesian optimization did a pretty good job at finding the hot spots out there.
12:11回到我的小胜利,基于事件的处理?所以我不想在4点开始的时候发现不是所有的前一个都在4点完成所以我在不完整的数据上运行。我也不想让我的过程在4点停止,因为所有的前一步都应该在凌晨3点完成。所以,我入侵了RapidMiner来搜索一个标记,上面写着“一切都完成了。你现在就可以开始了。”现在我提前15分钟跑了,还是15分钟之类的。只是基于事件的触发器的快速快照,在顶部。它只是一个循环,说我要做多少次这个测试?我使用60只是基于经验证据。然后这里有一个子进程,如果它运行超过60次,它会抛出一个错误并给我发送一封电子邮件。 So I’ve got noticed that things didn’t perform the way they should. At the bottom, I have a SQL query that looks for the token I’m looking for that says everything is done with a time stamp on it, and I wrap that up with Extract Performance, one of the native operators, and I say, “Is this binary condition met or not? No. Exit.” Trip everything else down line. So what’s next, right? One VM, 200 forecasts, eight hours. This part’s in grey. I haven’t done it yet. I’m going to do it in the next week or two. The idea is to take this process the same way that I handled the hyperparameters, the grid searching, and to split it into six mutually exclusive pieces and hammer each of the VMs. So not everyone’s going to think throwing more cores at it is the sexiest solution, but that’s how I’m going to do it right now.
13:54最后一件事。我们使用Facebook的盈利模型进行时间序列预测。如果你仔细阅读get条目了解它们在哪里,很可能会在短期内释放出来你可以传递函数,一个true/false语句,表示"我想让你做蒙特卡罗不确定性抽样"在最后的图上画不确定区间是一种昂贵的计算。这里不需要不确定区间。我喜欢把它关掉。我可以通过下载源代码并注释掉该部分来做到这一点,但我不希望它必须维护代码。我记得在早些时候的一个主题会议上,有人说维护代码不是最有趣的事情。我将等待Facebook推出新版本你可以简单地传递一个假值来进行蒙特卡罗模拟。重要的收获是你从1.3小时的运行时间变成了27分钟。 So that’s where the gains and run times come from. Lastly, Michael from Forester said something about using optimization as a skill set on top of prediction, right? It’s a complementary skill set. That’s where we’re going next. Optimization problems really is the call-out there. And what was it I wanted to say that– saying that he a funny comment this morning. Use math to spend cash, something like that. That’s what we’re going to do there.
15:25我想结束一下给大家一些时间提问关于RapidMiner是如何帮助我的。这是一个低代码的界面,对吧?所以如果你做得对,你不需要任何代码。如果您采用我在这里所做的方法,您仍然可以获得快速开发和快速测试。显然,我们与脚本语言集成在一起。它非常适合跨系统编排。我们有服务器,所以我们做所有的服务器端。我不用把笔记本电脑绑好几个小时。这都是在服务器端并行执行的,对吧?最后一件事是基于事件的hack,我把它放在那里,让我的工作早点开始。 So that’s how we achieve the goal. Highly accurate, highly scalable demand forecasts with the problem of shared resources are limited. And my peer and partner data scientists are spinning up their own projects and gobbling up all those resources as we speak. So the solution is creative thinking to keep the footprint small. I went faster than I planned, so.